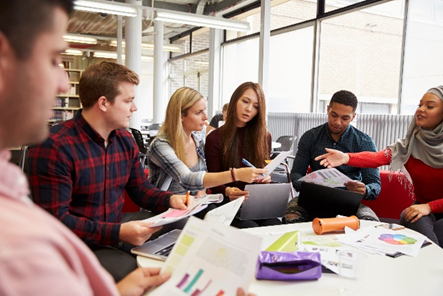
Historically, crowdsourcing has played an important role in certain fields of scientific research. Wildlife biologists often rely on members of the public to monitor animal populations. Using backyard telescopes, amateur astronomers provide images and measurements that lead to important discoveries about the universe. And many meteorologists use data collected by citizen scientists to study weather conditions and patterns.
Now, thanks largely to advances in computing, researchers in computational biology and data science are harnessing the power of the masses and making discoveries that provide valuable insights into human health.
Tackling Data on Complex Diseases
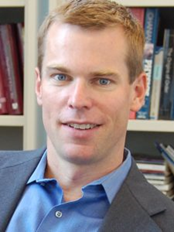
Credit: UCSD.
Trey Ideker, Ph.D., a professor of medicine at the University of California, San Diego (UCSD), has used crowdsourcing in his graduate-level bioinformatics classes to analyze results from genome-wide association studies. These studies allow researchers to identify particular gene variations that are linked with a disease or another trait.
Some diseases are triggered by single gene mutations or changes, but most conditions are much more complex. A combination of gene variations as well as environmental and other factors influence disease development.
“Common diseases like diabetes, cancer, heart disease, and neurological and psychiatric disorders have hundreds or even thousands of genes that are contributing to them,” Dr. Ideker says. “When you have diseases that involve so many genes, and the massive amount of data that’s associated with those genes, it’s hard to find connections.”
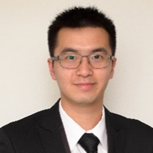
Students in Dr. Ideker’s class applied a classroom approach to crowdsourcing for a project on schizophrenia. The challenge was to develop computer algorithms that could generate a ranked list of 100 genes associated with schizophrenia. Students were given data on gene variants from more than 51,000 individuals.
“Setting this up as a competition pushed the students to explore different methods,” says UCSD graduate student Samson Fong, the teaching assistant for the class. “In a regular lab setting, you might have one or two people working on a problem. In this case, we allowed the students to develop eight or nine different approaches, which we could compare side by side. We learned much more about which methods worked and which didn’t.”
Dr. Ideker adds that running a competition in a classroom setting, rather than within the scientific community, encouraged collaboration as well as competition.
Fong agrees, noting that students learned a lot from each other. Additionally, they were guided to take their projects in different directions, to ensure a wider range of solutions. He explains that because students worked on teams instead of individually, they tackled much more complex problems than they could have on their own.
Advancing Science with a Combination of Computational Methods
The winning method from the competition led to a computational approach, called Network-Assisted Genomic Association, published in iScience. This approach outperformed other methods in identifying known disease genes and in how well the results from the analysis could be replicated. Dr. Ideker has already used the competition framework in another class to develop algorithms related to computational challenges in structural biology.
“This is a field that’s evolving so quickly that for most problems, there is unlikely to be only one computational method that will work,” Dr. Ideker says. “With this classroom approach, we see how the research can benefit from sampling a bouquet of different ideas. You’re pushing science forward in a really nice way.”
Dr. Ideker’s work is supported by NIGMS grant P41GM103504.