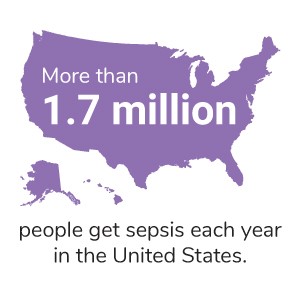
Sepsis is a serious medical condition caused by an overwhelming response to infection that damages tissues and organs. It’s unpredictable, progresses quickly, can strike anyone, and is a leading cause of hospital-related deaths. In the U.S. alone, nearly 270,000 people die each year from sepsis. Those who survive sepsis often end up in the hospital again, and some have long-term health complications. Early treatment is key for many patients to survive sepsis, yet doctors can’t easily diagnose it because it’s so complex and each patient is different.
Despite decades of research, sepsis remains a poorly understood condition with limited diagnostic tools and treatment. To tackle these obstacles, scientists Vincent Liu, Christopher Seymour, and Hallie Prescott have started using a “big data” approach, which relies on complex computer programs to sift through huge amounts of information. In this case, the computers analyze data such as demographic information, vital signs, and routine blood tests in the electronic health records of sepsis patients. The goal is to find patterns in the data that might help doctors understand, predict, and treat sepsis more effectively.
Machine Learning
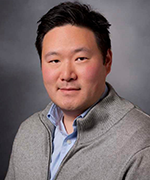
Vincent Liu, M.D. , of Kaiser Permanente Division of Research in Oakland, California, uses his Maximizing Investigators’ Research Award (MIRA) to look for signs that might identify patients at risk of developing sepsis even before they check into emergency departments. It turns out, he says, that as many as half of the people who develop sepsis had visited a doctor within a week prior to their hospital admission. This suggests that certain signs of illness recorded in electronic health records could provide a clue about which patients are at risk for sepsis. To identify these “pre-septic” signals, Dr. Liu uses machine learning, in which computers analyze vast amounts of patient data to reveal new insights and recognize patterns that would be impossible for humans to detect.
Dr. Liu also uses machine learning to estimate just how early a treatment for sepsis could be helpful. For example, because sepsis typically starts with a simple infection, antibiotics are a standard part of the treatment. But it wouldn’t be practical or safe for doctors to prescribe antibiotics to every patient at risk for sepsis. Bacteria are growing more and more resistant to antibiotics, and these antibiotics have side effects, such as killing patients’ beneficial bacteria and making them more susceptible to new infections in the future. Machine learning can help identify which patients would benefit the most and have the least risk from early administration of antibiotics. Electronic tools could routinely identify the highest-risk symptoms of infection so patients will know what signs should prompt them to get earlier medical attention.
Types of Sepsis
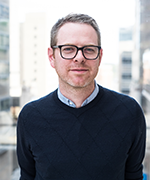
Christopher Seymour, M.D. , of the University of Pittsburgh Medical Center and his team are learning how sepsis patients differ from one another. His MIRA supports the Sepsis ENdotyping in Emergency Care (SENECA) project
. In SENECA, Dr. Seymour and his colleagues used computational tools to analyze the health records of more than 16,500 people who were diagnosed with sepsis within 6 hours of entering a hospital; diagnosis within 6 hours vastly improves survival rates from sepsis. The researchers focused on 29 different factors, such as age, sex, vital signs, routine blood tests and cell counts, concurrent diseases, and measures of organ function. They also used a group of more than 43,000 patients with a similar average age, sex ratio, lab results, and other clinical data.
The analysis revealed four distinct types, or phenotypes, of sepsis: alpha (α), beta (β), gamma (γ), and delta (δ), which differed in their clinical features, host response to infection, short- and long-term outcomes, and response to IV fluids. Dr. Seymour’s team continues to computationally scour through electronic health records to understand how current and future treatments may apply to these phenotypes. At the same time, the team is enrolling sepsis patients into a new study designed to investigate the biologic mechanisms underlying the four types. Someday, these phenotypes may help researchers design more effective clinical trials for sepsis, assist doctors in diagnosing the condition earlier, and optimize treatment procedures.
Health Consequences of Sepsis
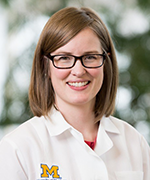
Hallie Prescott, M.D. , of the University of Michigan focuses on what happens to sepsis patients who survive initial hospitalization. She says that many people experience new physical illnesses or mental symptoms, and it’s common for patients to enter the hospital again within 3 months. Dr. Prescott wants to understand whether these negative consequences come directly from sepsis or from other illnesses the patient might already have. Doctors already know that certain pre-existing conditions such as autoimmune diseases make some patients more likely to develop sepsis in the first place.
Dr. Prescott recently co-chaired the international colloquium on understanding and enhancing sepsis survivorship . The group concluded that studies of sepsis usually focus on short-term survival, so there is little information on what happens to survivors in the months and years after sepsis. The group made several recommendations to advance research on sepsis survivorship. In the short term, it recommended that hospitals harmonize and share existing datasets to facilitate research. In the longer term, dedicated programs to study sepsis survivors are needed. This work will help doctors find and help patients who are more likely to have a worse prognosis after sepsis.
Dr. Liu’s research is supported by NIGMS grant R35GM128672, Dr. Seymour’s research is supported by R35GM11951904, and Dr. Prescott’s research is supported by K08GM115859.